Adding RIGOR to On-Farm Trials: Simple steps to data-driven decision making
Background: Why conduct on-farm trials?
Whether it’s a new variety, management technique, or application rate, on-farm trials are a producer’s first step toward evaluating performance. The best place to find out what works for a given situation and production system is on the farm where it will be used. No farmer should bet the farm on an unproven technique.
The way we demonstrate benefits to an operation is through on-farm testing. By utilizing a few key principles from the scientific domain, we can be more confident that the results of on-farm trials are valid and are not due to chance. This guide refers to five key principles of research design or RIGOR:
- Replication
- Include variation
- Guard against complexity
- Objective analysis
- Record keeping
Principles
Replication
It’s important to replicate experiments to guarantee that the introduced treatment or trial performs well across the farming operation, not just in one field. Even if a trial is repeated in the same location, the results might be different the following year. Replication affords confidence that the observed outcome is a result of the treatment and not chance. As the number of replicates increases, confidence increases in the observed outcome of the trial. If possible, replicates should be implemented in separate fields to improve confidence in results. Each replicate should be a complete block, meaning each replicate should contain all treatments and a control. Any trial needs a baseline reference for comparison, often called a “control.” Four to six complete blocks are considered sufficient for most on-farm research trials.
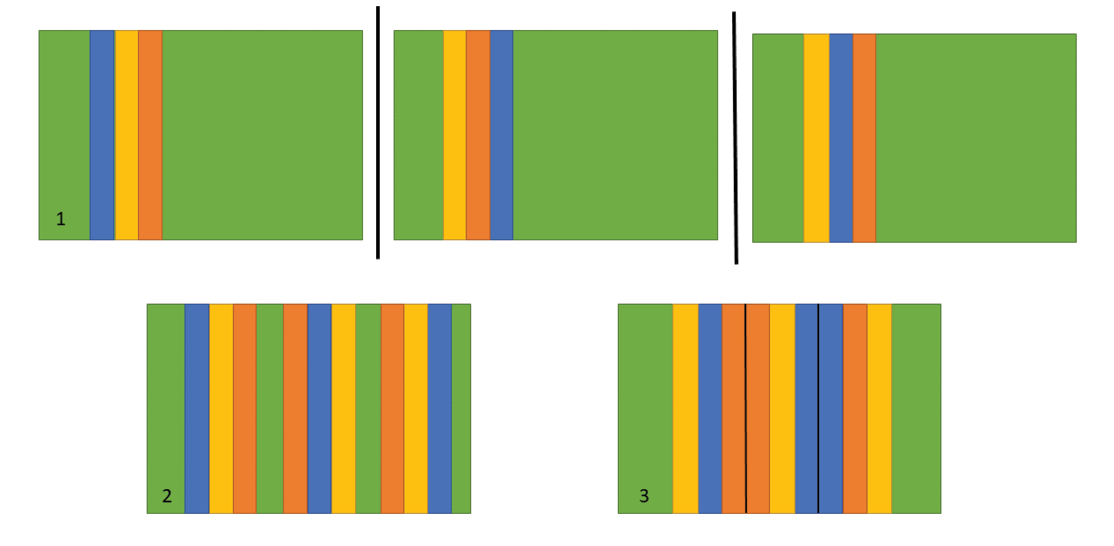
Include Variation
Care should be given during site selection, so the trial is not undermined by in-field variation. Farm fields typically have some level of variability, whether it be changing soil texture, low lying areas where water collects, or areas that consistently perform poorly or better relative to the entire field. To fully understand how the treatment will perform across all these areas, trial placement should encompass the range of potential conditions. It is best to implement a field trial at a right angle to the variation, such that it is not disproportionately represented in one of the treatments. However, in practice, choosing sites where each replicated group of treatments, or block, are completely homogenous is not always possible.

Guard against complexity
Factor: A variable under the control of the experimenter. Factors are conditions applied to an experimental unit.
Alter only one factor at a time. Resist the temptation to over complicate the trial by seeking to address multiple questions simultaneously. For every additional question, the number of required strip plots increases exponentially. Results from the trial may also be confounded and not reliable. Consider implementing separate trials for each question if multiple questions are of interest. Complete block designs have all variations (e.g. rates, levels) of a factor represented in each replicate. Altering more than one factor may confound results unless addressed in the analysis. There are statistical tests for considering more than one factor and the associated interactions, but this experimental design and analysis should be done with expert guidance. A split-plot experimental design may be appropriate when considering how two factors interact; each replicate should contain all combinations of the two variables.
Put it to practice
As a producer’s information needs increase, so does the complexity of analysis and number of trial strips or plots needed for proper replication. One factor trialed at four rates, for instance seeding rate, can require quite a few strips. If this hypothetical trial was replicated in three fields, it would require 12 strips. If additional questions or factors are added to the trial design, for instance a starter fertilizer application rate, the required number of strips increases very rapidly from 12 to 36 (4 seeding rates * 3 fertilizer rates = 12 combinations * 3 replicates = 36 strips). This example illustrates how added complexity in trial design can quickly increase the space and resources needed (4 seeding rates * 3 replicates = 12 strips in addition to 3 fertilizer rates * 3 replicates = 9 strips, totaling 21 strips).
Objective Analysis
Don’t let bias pre-determine the trial result. When it comes time to evaluate the data, it’s best to not have a preferred treatment. One way to avoid the potential for bias is to use a simple coding scheme for each treatment (1A, 1B, 1C). In this manner, an objective conclusion can be obtained as results are tallied because the treatment applied is not identified.
However, it’s important to record which code is associated with which treatment so that results can be traced and decoded once the trial has concluded. Decide beforehand what data need to be collected to answer the research question and let the results rest on those data. Specifically identify what metric(s) will be used to compare treatments, and what method will be used to obtain the metric. For example, if yield is the metric to be used, ensure that yield can be independently and reliably collected for each strip to make a fair comparison. Subjective metrics such as crop health, appearance, or “greenness” can be much harder to quantify and evaluate objectively.
Record Keeping
Life is busy and farm life moves quickly. It can be easy to forget eight, or even two, months prior. Because stakes and flags can easily become unreadable or lost over a season, draw a map of trial locations with treatment strips labeled accordingly. Consider counting rows for each treatment so that treatments are not lost in the field. Also include a description of each treatment, such as the product name or rate. Without such information, results will be difficult to interpret. While ongoing notes or observations may not factor into the final analysis, this information can also be documented as it may help inform future decision making. To conduct a valid analysis of the data, keep information on each strip separately. Do not combine data from treatment replicates to make an average. Data from each block should be kept separate for analysis.
Best Practices
Site selection
Site selection for on-farm trials will likely be a personal decision based on the operation. However, there are some guiding principles that strengthen the validity of results. Natural variation in and among fields, such as slope or soil texture, is ultimately unavoidable. As mentioned earlier, including such variability can improve the rigor of the trial.
Ideally, each site should have a similar range of variability due to the same underlying causes and be managed with the same practices to isolate the effects of the treatment. This could be achieved by selecting three neighboring fields, which likely have similar soil textures; will receive the same precipitation; and be planted, treated, and harvested around the same window of time.
Avoid trial locations near field edge as these areas are often not representative of the larger field. These areas are more susceptible to the outside effects of neighboring fields, trees lines, deer grazing, spray drift, or any number of other yield-reducing factors. In irregularly shaped fields, consistent sizing of strips or plots is less important than consistent management.
Strip trials
The most basic form of trial is a split-field design: The field is divided in half and a single treatment applied to only one side while the other side acts as a control. While the simplicity is appealing, it may not be feasible to accurately divide natural variation between the two halves, meaning that any conclusions drawn from this approach may not be valid. A strip trial is a field trial design where treatments are applied across the field in adjacent strips. Using strip trials increases the likelihood that variation is equally represented in all treatments. Prior yield maps, soil surveys, and historical knowledge of the field can all be informative in identifying underlying variation that should be considered when assigning the location of strips.
Good trial design assumes that all aspects, including variation, are approximately equal aside from the applied treatment. Thus, other non-trialed inputs should be applied uniformly across strips; unless the focus of the trial, variable rate applications should be avoided within the treatment strips. Any trial needs a reference for comparison, often called a “control.” In a strip trial, the control can simply be the rest of the field. For a more accurate representation of within-field variation, the control should be included as an untreated strip within the block of applied treatments.

Comparing treatments
It wouldn’t be fair to compare the best outcome of one practice to the worst outcome of another. An objective evaluation requires comparison of the most probable outcomes for treatment A and B. Trials without replication have greater challenges quantifying the true difference between treatments A and B, referred to as “effect size.” Identifying the effect size reduces the likelihood that spurious results will be accepted as legitimate and therefore improves the objectivity of the comparison. Although the ranges in observed values from treatments A and B may overlap, a true difference requires a significant difference in the midpoint of these ranges. As the number of replicates increases, a more accurate estimation of the true average is possible. True differences are established scientifically based on Least Significant Difference (LSD). LSD establishes the minimum difference between treatments that should be considered ‘significant’ and could be considered reliable.
Statistical significance is a way of attributing a measure of reliability in test results. Reliability is usually measured relative to levels of confidence, most commonly 95 percent in agricultural research. Analyses carried out at the 95 percent confidence level indicate a 5 percent likelihood of misattributing a difference between treatments when the result is due to chance.
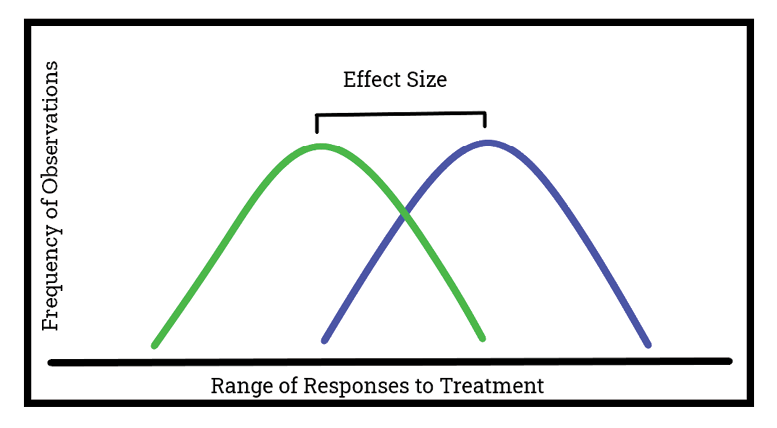
Consider a trial with three treatments that has been replicated five times, where the metric of interest is yield. The average yields for each treatment are 57.4 bu/ac for treatment A, 62.0 bu/ac for treatment B, and 48.2 bu/ac for treatment C. The calculated LSD is 10.6 bu/ac. Therefore, in order to be considered a statistically significant difference in yield, the difference between these averages must exceed 10.6. Although treatment B had the highest yield, it was not significantly different from treatment A. This means, with 95 percent certainty, either treatment would be expected to produce comparable yields. However, because the difference between treatment B exceeds that of treatment C by greater than 10.6, there is a less than 5 percent chance of incorrectly concluding there is no difference given the data.
Replicate |
Treatment A |
Treatment B |
Treatment C |
---|---|---|---|
One |
70 |
61 |
50 |
Two |
51 |
65 |
42 |
Three |
62 |
83 |
60 |
Four |
45 |
43 |
44 |
Five |
59 |
58 |
45 |
Average |
57 (bu/ac) |
62 (bu/ac) |
48 (bu/ac) |
This table displays how on-farm trial data would be organized if we measured yield response to 3 treatments (A, B, C) which were replicated 5 times (replicate 1-5) and measured, allowing us to calculate the average bu/ac yield response for each treatment.
Put it to practice
Why not just compare one replicate? Why is statistical significance more valid than averages?
The answer to both questions can be illustrated using the above example. If only one replicate were included, more than half of the trials would have indicated Treatment A was the best performer. However, based on the data from five replicates, the reality is that Treatment A wouldn’t perform any better than Treatment C in a normal year. Comparing only averages fails to account for how variable the data are. Combining all the data to make an average is akin to having only one replicate as the test loses the ability to consider the variation in the data.
Online calculators can be used to determine where chance ends, and true difference begins, assisting with the calculation of statistical significance. One application that may be useful is the University of Lincoln Nebraska FarmStat tool. The tool enables anyone with on-farm trial data to run simple statistical tests. Read the instructions and this manual carefully to ensure you are collecting and inputting quality data into the application.
Access the University of Lincoln Nebraska online statistics tool, FarmStat, and download user instructions prior to design and data collection.
Conclusion
In summary, on-farm trials allow farmers to generate their own data for decision making within their unique production system. Trials can also provide useful knowledge on implementation of new management techniques and experience with new products.
When applied appropriately, a few simple techniques can increase the RIGOR of on-farm trials. Natural in-field variation is often greater than the variation induced by applied experimental treatments and should be adequately included in trials. Objective assessment of trials results should include an unbiased review of treatment outcomes. Trial replication will greatly improve the overall accuracy of the result, while reducing the opportunity for erroneous conclusions.
Acknowledgements: This work was supported by USDA-NIFA 2022-68008-36356.
P3930 (10-23)
By Mark J. Hill, Extension Associate, Wildlife, Fisheries, and Aquaculture; Beth Baker, PhD, Associate Extension Professor, Wildlife, Fisheries, and Aquaculture; and Joby M. Czarnecki, PhD, Associate Research Professor, Geosystems Research Institute.
The Mississippi State University Extension Service is working to ensure all web content is accessible to all users. If you need assistance accessing any of our content, please email the webteam or call 662-325-2262.